Cerebral aneurysm rupture status classification using statistical and machine learning methods
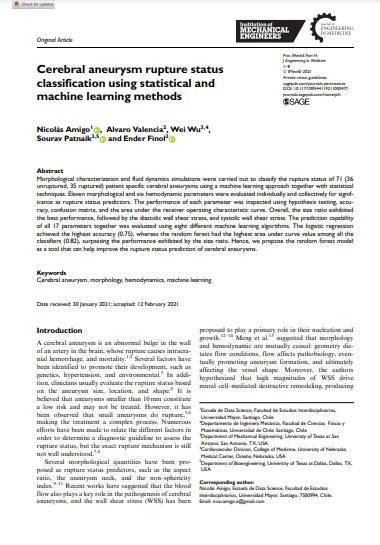
Fecha
2021-04-24Autor
Valencia, Alvaro
Wu, Wei
Patnaik, Sourav
Finol, Ender
Amigo, Nicolas [Univ Mayor, Fac Estudios Interdisciplinarios, Escuela Data Sci, Chile]
Ubicación geográfica
Notas
HERRAMIENTAS
Resumen
Morphological characterization and fluid dynamics simulations were carried out to classify the rupture status of 71 (36 unruptured, 35 ruptured) patient specific cerebral aneurysms using a machine learning approach together with statistical techniques. Eleven morphological and six hemodynamic parameters were evaluated individually and collectively for significance as rupture status predictors. The performance of each parameter was inspected using hypothesis testing, accuracy, confusion matrix, and the area under the receiver operating characteristic curve. Overall, the size ratio exhibited the best performance, followed by the diastolic wall shear stress, and systolic wall shear stress. The prediction capability of all 17 parameters together was evaluated using eight different machine learning algorithms. The logistic regression achieved the highest accuracy (0.75), whereas the random forest had the highest area under curve value among all the classifiers (0.82), surpassing the performance exhibited by the size ratio. Hence, we propose the random forest model as a tool that can help improve the rupture status prediction of cerebral aneurysms.
URI
https://repositorio.umayor.cl/xmlui/handle/sibum/9060https://doi.org/10.1177/09544119211000477
https://journals.sagepub.com/doi/10.1177/09544119211000477
Coleccion/es a la/s que pertenece:
Si usted es autor(a) de este documento y NO desea que su publicación tenga acceso público en este repositorio, por favor complete el formulario aquí.